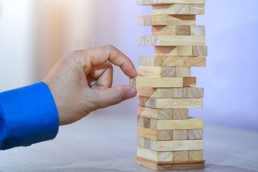
Model Your Customer
Strengthen Customer Engagement and Retention
David Haertzen - September 21, 2024
Would you like to better understand your customers? Customer knowledge can both help to improve revenues, reduce losses and avoid risks. A customer is broadly described as a party (person or organization) who is of interest to the enterprise. A customer model that identifies characteristics of your customers is a great way to achieve a level of understanding and achieve company goals. This article introduces customer modelling.
There are two broad categories of customer models: data models and analytical models. Data Models are representations, usually through graphical means, of facts, statistics, or items of information. Data models may be used to: understand data, communicate the structure of data and design data structures. In contrast, Analytical Models are representations of reality coupled with
algorithms that produce results such as: classifications, predictions, optimizations or recommendations.
Customer Data Model
This information can be organized into a high level data model for better understanding and easier management. This diagram shows the Shrewd Data high level Customer Data Model.
Examples of data for each topic in the customer data model include:
- Customer Identifiers: Account numbers, tax id numbers, drivers license numbers
- Demographics: Birthdate, age, gender, marital status, education level
- Measures: Net worth, income, revenue, credit score, Lifetime Customer Value (LCV)
- Behaviors: Social media, driving record, address changes
- Locations: Geo location (country, state, city, zip), district, telephone
- Psychographic: Opinions, values, sentiments, preferences, risk tolerance
- Transactions: Purchases, returns, payments, deposits, withdrawals
- Interactions: Service calls, web visits, abandoned cart, response to offers
- Products: Current products owned, products under consideration
These topics are the “tip of the iceberg”. A full 360 degree view of customer will be comprised of multiple data stores with multiple data sources.
Customer Analytical Models
Analytical models apply algorithms to data to: enable better understanding, make predictions or recommend decisions. Examples of customer analytical models include:
- Acquisition Model: A model that predicts the probability that a prospect will buy the company’s products or services.
- Cross-sell Model: A model that predicts the probability that an existing customer will buy additional products or services of a different type than currently bought. Goods are at the same level.
- Up-sell Model: A model that predicts the probability that an existing customer will buy an upgraded product or service.
- Attrition Model: A model that predicts the probability that an existing customer will stop purchasing the company’s products or services. This also known as a churn model.
- Value Model: A model that predicts a numeric value such as customer lifetime value (CLV) or value resulting from the sale of a specific product to a customer.
- Tone-Of-Voice Model: A model that identifies the most effective message for each targeted customer.
- Risk Model: A model that predicts potential negative activities by customers such as: fraud, money laundering, loan defaults, or excess service costs.
- Customer Segmentation Model: A model that assigns customers to groups with similar characteristics.
- Recommendation Engine: A model that provides advice on a near real-time basis – such as advice about offers that should be made to a customer or additional products to show to a customer.
- Look-alike Model: A model where the target-marketed group (e.g. for a marketing campaign, product offering etc.) is an expanded list of parties whose profiles look like the selected party.
Customer Model Conclusion
In conclusion, customer models are powerful tools that have the potential to impact an organization’s bottom line. You can learn more about this topic by studying additional articles and white papers provided on the Shrewd Data and other websites.